Score-based Causal Representation Learning
- (AAAI 2025 Tutorial) Causal Representation Learning
- (NeurIPS 2024) Linear Causal Representation Learning from Unknown Multi-node Interventions
TLDR:
- Linear transform, general causal models, unknown multi-node interventions. Same guarantees as single-node interventions!
- Hard interventions: element-wise identifiability up to scaling and perfect recovery of DAG.
- Soft interventions: identifiability up to ancestors
-
Requirement: multi-node interventions are diverse enough, specified as having a full-rank intervention signature matrix.
- (NeurIPS 2024) Sample Complexity of Interventional Causal Representation Learning
TLDR:
- Linear transform, general causal models, one single-node soft intervention per node.
- First sample complexity results for interventional CRL!
- Probably approximately correct (PAC)-identifiability via generic score estimators.
-
Specific sample complexity results for an RKHS-based score estimator.
- General Identifiability and Achievability for Causal Representation Learning (AISTATS 2024 - oral)
TLDR:
- General transform, general causal models, two single-node hard interventions per node suffice for element-wise identifiability (up to an intervible transform)!
- First provably correct algorithm for general transforms! Experiments with images confirm the scalability.
-
Benefits: do not require faithfulness assumption of causal discovery, and do not require to know which pair of environments intervene on the same node.
- Score-based Causal Representation Learning: Linear and General Transformations (under submission)
TLDR:
- Linear transform, general causal models, one single-node intervention per node.
- Hard interventions: element-wise identifiability up to scaling and perfect recovery of DAG.
- Soft interventions: identifiability up to ancestors. If the causal model is sufficiently nonlinear, then latent DAG is fully identified and latent variables are identified up to surrounding variables (shown to be a tight result)
-
General transform: reorganization of the AISTATS paper, with additional experiments.
- Score-based Causal Representation Learning with Interventions
=======================
Project summary:
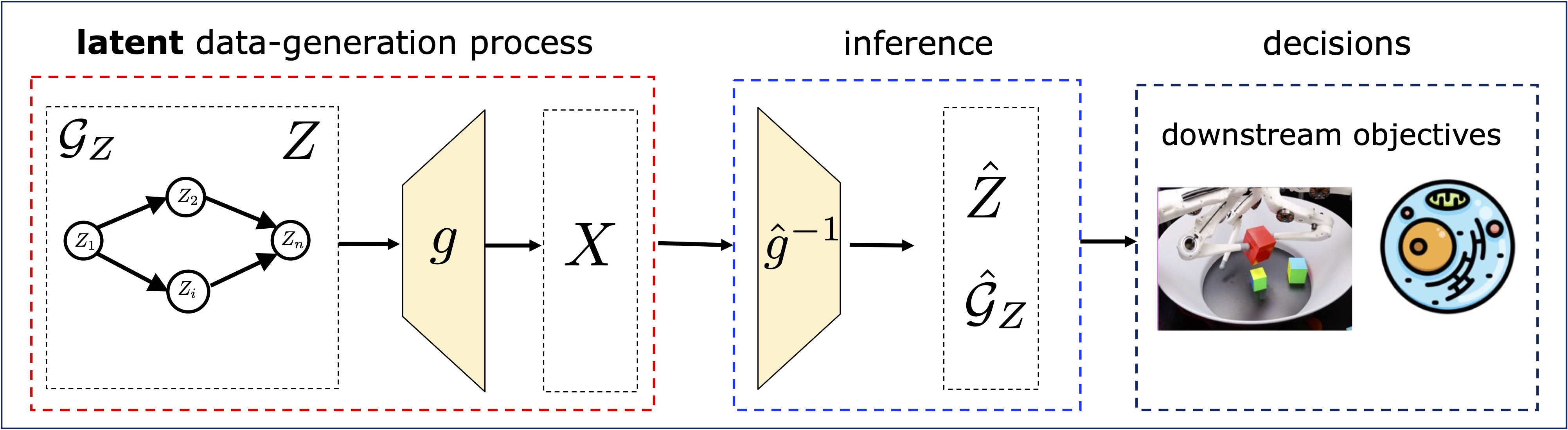
In causal representation learning (CRL), we consider a data-generating process in which the high-dimensional observations \(X\) are generated from low-dimensional, causaly-related variables \(Z\) through an unknown transformation \(g\) as \(X=g(Z)\). The causal relationships among the latent variables are captured by a directed acyclic graph (DAG) \({\cal{G}}_{Z}\) over \(Z\).
CRL is the process of inverting the data generation-process for using the observed data \(X\) and recovering (i) the causal structure \(\cal{G}_{Z}\) and (ii) the latent causal variables \(Z\). We focus on two central questions:
-
Identifiability: Determining the necessary and sufficient conditions under which \({\cal{G}}_{Z}\) and \(Z\) can be recovered. The scope of identifiability (e.g., perfect or partial) critically depends on the extent of information available about the data and the underlying data-generation process.
-
Achievability: Designing algorithms that can recover \(Z\) and \(\mathcal{G}_{Z}\), while maintaining identifiability guarantees. Note that identifiability results can be non-constructive as well, without specifying feasible algorithms. Hence, we make the distinction and aim to design practical algorithms for achieving constructive identifiability results.
Why is identifiability difficult? Identifiability is known to be impossible without additional supervision or sufficient statistical diversity among the samples of the observed data \(X\). For instance, for the true transform \(g\) and another invertible function \(a\), we have \((g \circ g^{-1})(X)=X\) and \((g \circ a^{-1}) \circ (a \circ g^{-1})(X)=X\). Hence, inverse transform \(g^{-1}\) cannot via ensuring reconstruction of \(X\). As an extreme simplification, linear mixing of independent Gaussian variables (i.e., a graph with no edge). Since Gaussians are rotation-invariant, we cannot distinguish between \(Z\) and \(R \circ Z\) for any rotation matrix \(R\).
Therefore, to ensure identifiability, we need to look for a reasonable combination of (i) assumptions on the data-generation (model class we consider), and (ii) richer observations.
Interventional CRL
In our work, we rely on interventions on the latent causal space to provide richer observations and sufficient statistical diversity to enable identifiability. Specifically, in addition to observational environment, we consider a set of given interventional environments, in which a subset of nodes are intervened in each. In this framework, we only use distribution level information, meaning that we use the interventions as a weak form of supervision via having access to only the pair of distributions before and after an intervention (as opposed to requiring pairs of observed and intervened samples). This allows us:
- model distribution shifts via changes in causal mechanisms
- contrast interventional vs. observational distributions
- if changes are sparse, gives a natural way to restrain the solution set

Ideally, one would prefer no restriction on intervention size, type, or knowledge. In our papers, we consider different settings, e.g. single-node vs. multi-node interventions and soft vs. hard interventions.
Score-based methodology
In our papers, we establish the connections between score functions (gradient of the log-density) and interventional distributions. Specifically, we show that differences in score functions across different environment pairs contain all the information about the latent DAG. Leveraging this property, we show that the data-generating process can be inverted by finding the inverse transform that minimizes the score differences in the latent space. Using this approach, we develop constructive proofs of identifiability and algorithms in various settings. To give an insight, there are two key properties of score functions that make this idea work. Denote \(s(z) = \nabla \log_z p(z)\) and \(s_X(x) = \nabla \log_x p(x)\) for observational distributions \(p(z)\) and \(p_X(x)\). Use superscript \(^m\) to denote corresponding definitions in interventional environment \({\cal E}^m\).
- Score differences are sparse: Consider a single-node intervention, e.g., node $i$ is intervened in \({\cal E}^m\). Then, the score difference function \(s(z) - s^m(z)\) will be sparse, with indices of non-zero entries exactly correspond to the parents of the intervened node. This implies that given access to all single-node interventions, changes in the score functions exactly give DAG structure!
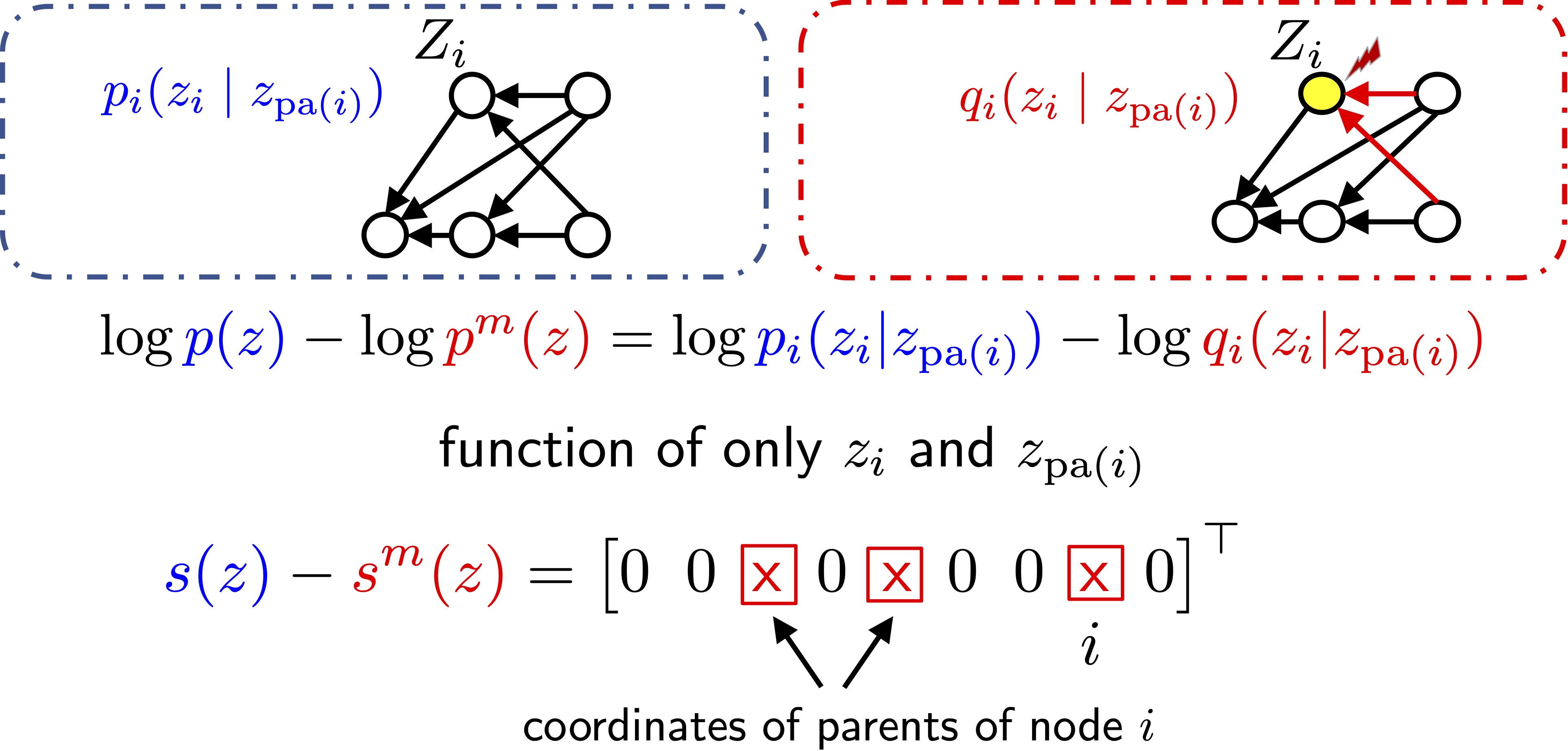
How we use this property to guide our learning of an inverse transform? Consider a candidate encoder $h$, and \let $\hat{Z} = h(X)$. Intuitively, we can use the sparsity of the true latent score differences to find the true encoder \(g^{-1}\), i.e., the estimated latent score differences cannot be sparser than the true score differences.
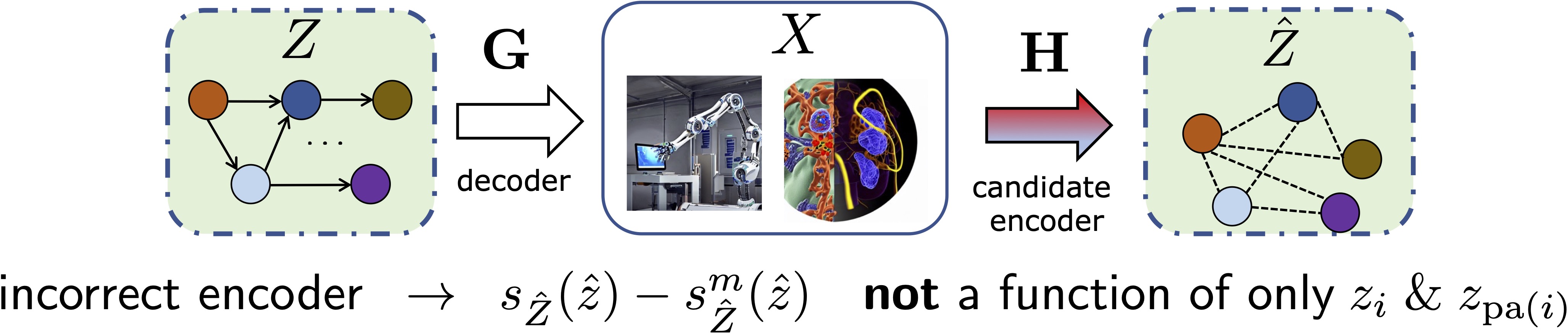
- Latent score differences can be computed from observed score differences: So far, all the nice properties of score functions above are for latent variables, since these properties stem from the causal relationships among the latents and the interventions. However, we have only access to observed \(X\) variables. In terms of pure identifiability objective, one can suggest computing the score functions of $\hat{Z}$ for every possible encoder \(h\), which is infeasible. Instead, we take a constructive approach and show that latent score differences can be computed from observed score differences using the Jacobian of \(h^{-1}\). Specifically, \(s_{\hat{Z}} ({\hat{z}}) - s_{\hat{Z}}^{m}({\hat{z}}) = J_{h^{-1}}({\hat{z}})^{\top} (s_{X} (x) - s_{X}^{m}(x))\).